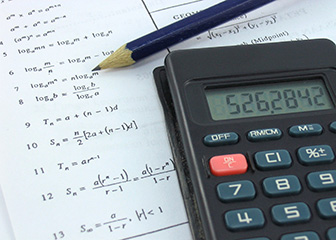
One of the major inclinations of the empirical researchers in the field of economics is to carry out research works, which involve time series data, so that they can capture the change in the nature of the variable(s) over a long period of time. Though for analysis with this kind of data, methodologies like vector auto regression, vector error correction, autoregressive conditional heteroscedasticity etc. are available in the field of econometrics, researchers relying on the regression technique try to analyze this type of data with regression method only.
However, while carrying out a regression based study on the time series data, several problems can arise, which can result in spurious regression. One of the major problems in this category is the presence of serial correlation or autocorrelation present in the set of independent variables. This is a serious problem, and in most of the cases, researchers overlook this particular aspect, and carry out the analysis, which in most of the cases are wrong. However, there is an easy way out to get rid of this problem, and the solution is to carry out the auxiliary regression, along with the original regression model. This auxiliary regression is not a very new solution to get rid of the problem of serial correlation, and it has been used in several contexts. Once the regression model is formulated and estimated, a set of additional regression models are needed to be designed among the independent variables only. Out of all those additional regression models, there will be some models, which will demonstrate higher regression coefficients than that of the original model. From those set of models, the variables are needed to be chosen, the standard errors of which are significantly high. Removal of those variables from the original model can result in removal of serial correlation.
To know more about the different aspects of auxiliary regression, kindly browse through the pages of www.sampledissertations.com.
However, while carrying out a regression based study on the time series data, several problems can arise, which can result in spurious regression. One of the major problems in this category is the presence of serial correlation or autocorrelation present in the set of independent variables. This is a serious problem, and in most of the cases, researchers overlook this particular aspect, and carry out the analysis, which in most of the cases are wrong. However, there is an easy way out to get rid of this problem, and the solution is to carry out the auxiliary regression, along with the original regression model. This auxiliary regression is not a very new solution to get rid of the problem of serial correlation, and it has been used in several contexts. Once the regression model is formulated and estimated, a set of additional regression models are needed to be designed among the independent variables only. Out of all those additional regression models, there will be some models, which will demonstrate higher regression coefficients than that of the original model. From those set of models, the variables are needed to be chosen, the standard errors of which are significantly high. Removal of those variables from the original model can result in removal of serial correlation.
To know more about the different aspects of auxiliary regression, kindly browse through the pages of www.sampledissertations.com.